Recent News
EPA awards UNM $7M to support small water systems
May 29, 2025
Computer scientist wins Athlete of the Year Award for adaptive skiing technique
May 27, 2025
UNM to host first-ever residential nuclear engineering camp
May 20, 2025
UNM Regents' Scholar Rebecca Nez named 2025 Udall Scholar
May 15, 2025
News Archives
ECE graduate student proposes new way for solar forecasters to predict cloud cover
February 21, 2022 - Melanie Furber Fudge, UNM Center for Advanced Research Computing
A graduate student in The University of New Mexico Department of Electrical and Computer Engineering has designed a new way for solar forecasters to predict future cloud cover, which is a persistent problem in the renewable energy field.
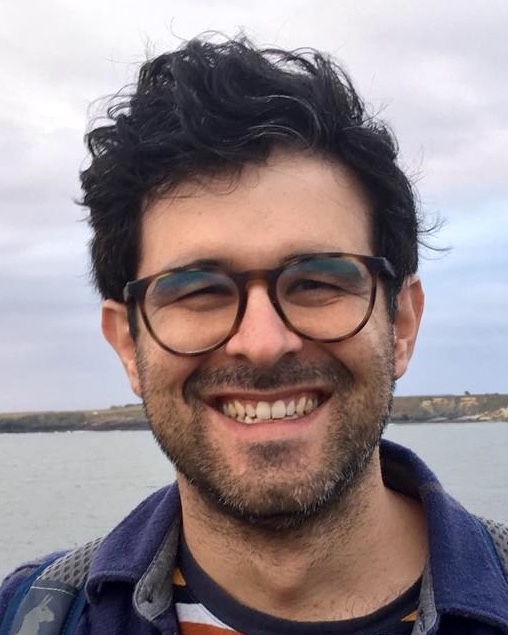
Guillermo Terrén-Serrano is a Ph.D. candidate working with advisor Manel Martínez-Ramón, a professor in the Department of Electrical and Computer Engineering, and according to Martinez-Ramón, the approach is innovative, multidisciplinary and built completely from scratch.
“I think that this work might have an impact in energy forecasting, which is very important right now and will continue to be very important in the future,” said Martínez-Ramón, who also holds the title of King Felipe VI Endowed Chair.
According to Terrén-Serrano, there is a paradoxical problem with renewable energy, which is generated by wind and sunlight, meaning that it is dependent upon the availability of wind and sunlight. Consequently, weather conditions, especially cloud cover, can affect the gathering of these renewable energies. Adverse weather conditions can severely impact renewable energy providers, who are prevented from connecting to power grids if they have a decrease in their energy production.
“The grid operators are limiting the ramp rates, [or] the drops in the energy you can send through the transmission lines. So, if there is a drop in your ramp rate, they are going to cancel you and not allow you to connect to the grids,” he said.
To solve this problem, Terrén-Serrano designed an end-to-end system to predict future cloud cover known as a clear sky index. By knowing when clouds will be obscuring the sun, providers can use batteries to compensate for the drop in solar energy, ensuring a steady energy load. This will prevent drops in their ramp rates and eliminate the possibility they will be barred from the power grid for violating ramp rate guidelines.
“What we are trying to do is increase renewable resources [by making it] possible for PV [photovoltaic] operators to connect their plants to the power grids without violating their ramp rates and without the risk of being unplugged,” he said.
To accomplish this, Terrén-Serrano and Martínez-Ramón determined that they would tackle the solar forecasting problem from a new angle.
The perspective is “different from other published papers that we know about,” said Martínez-Ramón.
In the past, solar forecasting has been based on algorithms that have been published in a computer journal or found in a software assistant repository which have been adapted to do solar forecasting.
“This creates a lot of problems, and we talk about this in the papers,” Terrén-Serrano said.
“We want to get rid of the concept of ‘black box’ in solar forecasting. By black box, we mean the use of an already-published technique, applied to the problem at hand, without modification or interpretation of the behavior of the machine in terms of the solar forecasting process,” Martínez-Ramón said.
The team’s multidisciplinary approach borrows concepts from fluid dynamics, atmospheric dynamics, physics and standard and non-standard image processing techniques, and combines these concepts with machine learning, kernel learning and deep learning.
This utilization of machine learning instead of end-to-end learning is very important, according to Terrén-Serrano. Their system, which he calls “image understanding,” is based on a sequence of images taken by a camera which follows the sun throughout the day. The machine learning algorithms he created then analyze the pictures to chart what is happening with the clouds. The result is solar forecasting of future cloud cover based on this analysis.
For example, think of a cloudy afternoon sky. The clouds in the distance look like they are barely moving, while the clouds that are near appear to move much faster. But this appearance is deceptive because it is based on our perspective. In reality, the clouds in the distance are moving quite fast.
One of the key components of Terrén-Serrano’s new system is that it analyzes each picture taken of the cloud formations pixel by pixel, obtains an accurate dimension of each pixel, and determines the actual velocity of the cloud formation. Martínez-Ramón said what Terrén-Serrano created “is a methodology to undo the perspective so all the clouds [in the transformed image] are moving at the real speed.”
He said it is of notable importance that Terrén-Serrano developed an integral system from end to end that gathers the data from a camera and a sensor and provides an accurate solar power forecast.
“All the elements of the system are innovative, including the data processing, physical models, information retrieval and machine learning applied to this information,” said Martínez-Ramón. “Everything has been developed from two cameras mounted on a servo system to follow the sun, some electronics developed by us and a small computer.”
Martínez-Ramón said the system is described in Terrén-Serrano’s doctoral dissertation, which contains than 250 pages of publishable work and is considerably longer and more detailed than typical dissertations.
When Terrén-Serrano began designing his system for his dissertation, he was told that he would never get the wind velocity vector right. He accepted this challenge and succeeded with being able to establish an accurate wind velocity vector.
His paper on geospatial perspective reprojection is currently under review for publication and explains how he achieved his results. And this is not the only paper to have come out of Terrén-Serrano’s research. He has written 12 other papers about his forecasting system which have been published, presented at conferences, or are under review for publication.
Terrén-Serrano credits UNM’s Center for Advanced Research Computing (CARC) with giving him the capability to build his new solar forecasting system. Both Terrén-Serrano and Martínez-Ramón acknowledge that his work might have been impossible, or at least at lot more difficult, without CARC. Both express their gratitude to CARC not just for the computer systems, but also the help they provide the students.
“We are really, really thankful for CARC because [they] do a great job assisting the students with their work when they have trouble,” Martínez-Ramón said.
“We had ideas of how to do this work and we talked about this program, [but it was] CARC that gave me the trackability,” Terrén-Serrano said. “I could get all the data and handle it in a way that is efficient.”
Utilizing CARC’s central processing units (CPUs) and graphics processing units (GPUs), he was able to research specific areas from the sky imagers and tie those areas into other processes such as cloud tracking, cloud detection, image processing and solar forecasting. He was then able to bring it together with machine learning and artificial neural networks.
“With CARC’s systems I can use kernel learning or deep learning, which is computed in the GPUs, so I have all of these possibilities technologically of doing an improvement in these areas of research,” he said.
Martínez-Ramón said he has enjoyed working with Terrén-Serrano in this new discovery.
“It is Guillermo’s work; I am just the advisor. I had a lot of fun working with him, a lot. Because there is a lot of theory and a lot of new things.”
Terrén-Serrano is currently finalizing his dissertation in anticipation of a spring 2022 graduation.